Is the Healthcare System Stable?
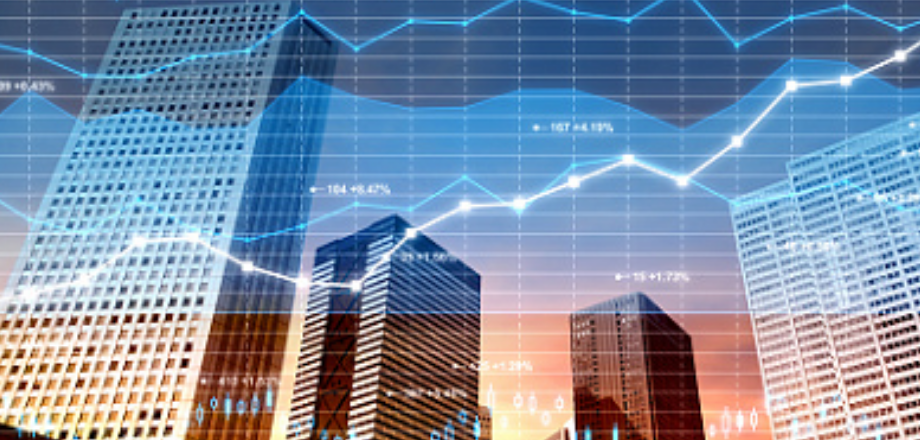
By Rocco Perla
Twenty years ago, the Dartmouth Atlas provided a first glimpse into the glaring variation in how medical resources are distributed and used in the United States. Why do some primary care physicians order more than twice as many CT scans as their colleagues in the same practice? Why is the cost of a hip replacement three times more expensive in one region in the U.S. than another? Not only did the Atlas signal alarming variation in cost, quality and utilization across the country, it demonstrated the health system was not stable – that is, the cost or quality for a procedure in one health system did not predict the cost or quality in another system. But what specifically is the relationship between stability and variation? And why does it matter?
To know if a system is stable, we first have to understand variation. There is variation in all aspects of our lives: our household expenses, behaviors, stress levels, and commute times. There is variation among institutions. Test scores for students in different schools vary from year-to-year. Crime rates in different communities change from month-to-month. Profit margins differ between companies from quarter-to-quarter. Success rates for the same surgical procedures vary between different hospitals. We constantly make decisions in our daily lives around this variation: Is it time to change schools? Is the crime rate getting worse? Should I abandon the bus and start taking the train?
In 1931, Walter Shewhart helped us understand that variation can be stable or unstable over time – a clue as to whether we should do something about it. If the variation is similar to past experience, then the system is stable (relatively predictable from point-to-point); if the variation is all over the place and past experience provides no guidance about how the system will perform in the future, then the system is unstable. Unusual patterns of variation merit examination and/or action, while random variation that is similar to what has been experienced in the past likely do not require action. Mistakes – overreacting and under-reacting to data – play out millions of times each day across our knowledge economy, at a significant cost. There are two mistakes we can make around variation in data:
- Assume the variation is not random and is due to some special cause, when in fact it is random(i.e., part of the normal fluctuation of data over time). Calling a special meeting to discuss why this month’s patient satisfaction score is lower than last month’s score, even though it’s not actually that different from past performance, is an example of this type of mistake.
- Assume the variation is random, when in fact it is not. For example, one surgeon has an unusually high infection rate this month, but it’s missed because the data are analyzed by aggregating all surgeons together each month which masks the performance of this one surgeon.
Failure to understand variation causes confusion, inhibits learning, produces poorer outcomes, and demoralizes leaders and workforces. And if we do not know if the data are stable over time, we cannot know what to expect in the future. Shewhart didn’t stop with just a theory and a framework, he developed a simple tool— called the control chart—to distinguish between variation that was random (i.e., due to “common causes”) and variation that was non-random (i.e., due to “special causes”). The control chart presents data over time (e.g., week, month or quarter) and includes 3 lines: the center line which is the average of all the data points, and upper and lower limits based on the average and an estimate of the standard error. All three lines come together define the parameters of a process moving forward in time (see Figure 1). By analyzing data over time using this approach, we can assess if the variation is random or not. One sign of non-random variation would be a single data point below or above the limits.
Figure 1 represents a control chart looking at the number of unique patients who screen positive for an unmet social need in seven states across 21 clinical sites. This information is critical for health systems to understand—in the context of health reform—given that 60% of the modifiable factors of health are linked to social determinants. What we learn from this data is that the screen positive rate is stable over the last 28-week period. Based on this data, if conditions remain the same, we can predict the average screen positive rate will continue to be 300, going as low as 205 or as high as 395 – the upper and lower limits. This is the predictable output of the system, which can help determine resource needs and staffing. As tempting as it might be to react to the highest value at week 41, that would be equivalent to explaining why you flipped a coin and got heads – just random variation.
Today, for all the talk and focus on big-data, data-driven decision-making, and business intelligence, uptake of Shewhart’s framework and tool for understanding variation has not been proportional to its potential for impact. We have spent decades and billions of dollars implementing and evaluating interventions not knowing if our experiments are occurring in stable or unstable systems – and therefore misunderstanding or over/underestimating their impact.
This one approach to analysis can be transformational. Take the stability of a clinical process in our healthcare system. Until recently, performing coronary artery bypass surgery was an unstable system in which outcomes (including death) varied depending on the surgeon or the hospital where the surgery was performed. But no one knew it. Only by studying variation over time for this procedure and recognizing its association with specific surgeons and hospitals were stable outcomes achieved in states like California and New York – resulting in dramatic decreases in mortality rates to less than 2%. As one researcher recently observed, “It is now almost impossible to identify a surgeon or hospital in either state that is better or worse than other surgeons or hospitals.”
So why hasn’t Shewhart’s approach become a dominant analytic framework in the U.S. and what are the consequences? My colleagues and I attempt to answer this question in “Understanding Variation – 26 Years Later,” a recent article in Quality Progress. In this piece, we demonstrate through a series of examples the distortion that occurs when data (especially those derived from large publicly available sources) are interpreted – and decisions made —without understanding if the outcomes of the system are stable.
For example, every year, the Bureau of Labor Statistics (BLS) analyzes fatal work injury data and reports a color-coded map showing whether a state’s number of fatal work injuries increased, decreased or stayed the same from the previous year. We created the control chart in Figure 2 using BLS data from 1992-2013, but we also added the year-to-year assessment you get from the color-coded map approach that indicates if things are getting better or worse. The control chart shows that from 1992 – 2010 the system was stable – that is, the average fatal work injury rate was 4 per 100,000 people and could fluctuate between a low of 1.7 and a high of 6.4. For 18 years, that system was stable without a single value exceeding the upper limit – until 2011. In 2012, North Dakota officials grew concerned about the increased frequency of fatal injuries, which some attributed to an increased number of workers with riskier jobs in sectors such as the oil industry, as the energy sector grew. Unlike the Shewhart chart in Figure 2, which provides a system view of all data and reveals the upper and lower limits of North Dakota’s fatal injuries over time — the color-coded map limits the analysis to whether conditions are getting better or worse from the prior year. And in 2013 the rate is lower than 2012, but are things really better? That’s what you would think from the year-to-year comparison, but from the control chart we see 2013 still exceeds the upper limit. The system is not stable and we need to know why. What should a worker in North Dakota think, or the family member of a worker who was killed in 2012?
The opportunities to improve how we learn from and act on data using Shewhart’s framework are limitless. For example, the USDA recently reported that food insecurity across U.S. households declined significantly from 14.0% in 2014 to 12.7% in 2015. Their website states that “the cumulative decline from 2011 (14.9%) to 2014 (14.0%) was statistically significant, and that downward trend continued in 2015.” But the real story is that food insecurity was stable between 1995 – 2007 (first center line = 11.1%), but in 2008 we see an upward shift that stabilizes at rate of 14.5% for seven years (second center line). This means the “new normal” for food insecure households increased by 30.6% in a single year. That cumulative decline from 2011 – 2014 was not a signal of non-random variation in the control chart, and even though it was directionally desirable – nothing was changing fundamentally. It is not until 2015 that we get a data point outside what we would expect – below the lower limit. The greatest learning comes from studying the two non-random patterns we see in the data: the shift that occurs from 2007 to 2008 and the most recent data point in 2015. These are signs that something unusual is occurring and it makes economic sense to invest time and resources to study these periods – beyond any others. What can we learn from the signal in 2015? Are there certain policies or programs that led to this result that we need to keep investing in to get us back to pre-recession levels? These are the questions we must ask.
An understanding of variation using Shewhart’s framework can unlock some of our most fundamental public policy challenges by enabling data to reveal where we should focus our finite time, dollars, and research capacity. This approach has three levels of impact.
First, it’s a method that people intuitively understand – whether you’re an oil refinery worker in North Dakota, a coronary artery bypass graph patient in California or a hungry family in the U.S., it is easy to visualize a stable process and understand limits.
Second, the framework allows decision-makers and leaders to minimize risk of over and under-reacting to data.
Lastly, this approach can guide the design of systems, which are effectively the accumulated effects of decisions that are made over time.
Some might think that all this is simply an issue of methods but it is not; it is about the way we construct meaning from experience.